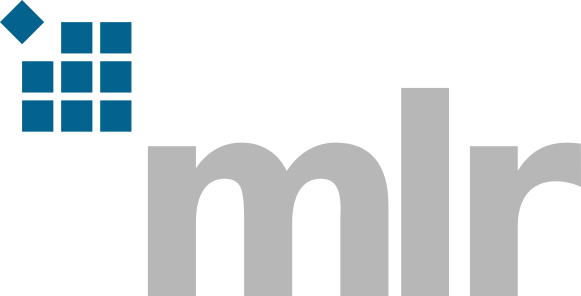
Hyperparameter Tuning with Covariance Matrix Adaptation Evolution Strategy
Source:R/TunerBatchCmaes.R
mlr_tuners_cmaes.Rd
Subclass for Covariance Matrix Adaptation Evolution Strategy (CMA-ES).
Calls adagio::pureCMAES()
from package adagio.
Control Parameters
start_values
character(1)
Createrandom
start values or based oncenter
of search space? In the latter case, it is the center of the parameters before a trafo is applied.
For the meaning of the control parameters, see adagio::pureCMAES()
.
Note that we have removed all control parameters which refer to the termination of the algorithm and where our terminators allow to obtain the same behavior.
Progress Bars
$optimize()
supports progress bars via the package progressr
combined with a bbotk::Terminator. Simply wrap the function in
progressr::with_progress()
to enable them. We recommend to use package
progress as backend; enable with progressr::handlers("progress")
.
Logging
All Tuners use a logger (as implemented in lgr) from package
bbotk.
Use lgr::get_logger("bbotk")
to access and control the logger.
Optimizer
This Tuner is based on bbotk::OptimizerBatchCmaes which can be applied on any black box optimization problem. See also the documentation of bbotk.
Resources
There are several sections about hyperparameter optimization in the mlr3book.
Getting started with hyperparameter optimization.
An overview of all tuners can be found on our website.
Tune a support vector machine on the Sonar data set.
Learn about tuning spaces.
Estimate the model performance with nested resampling.
Learn about multi-objective optimization.
Simultaneously optimize hyperparameters and use early stopping with XGBoost.
Automate the tuning.
The gallery features a collection of case studies and demos about optimization.
Learn more advanced methods with the Practical Tuning Series.
Learn about hotstarting models.
Run the default hyperparameter configuration of learners as a baseline.
Use the Hyperband optimizer with different budget parameters.
The cheatsheet summarizes the most important functions of mlr3tuning.
Super classes
mlr3tuning::Tuner
-> mlr3tuning::TunerBatch
-> mlr3tuning::TunerBatchFromOptimizerBatch
-> TunerBatchCmaes
Examples
# Hyperparameter Optimization
# load learner and set search space
learner = lrn("classif.rpart",
cp = to_tune(1e-04, 1e-1, logscale = TRUE),
minsplit = to_tune(p_dbl(2, 128, trafo = as.integer)),
minbucket = to_tune(p_dbl(1, 64, trafo = as.integer))
)
# run hyperparameter tuning on the Palmer Penguins data set
instance = tune(
tuner = tnr("cmaes"),
task = tsk("penguins"),
learner = learner,
resampling = rsmp("holdout"),
measure = msr("classif.ce"),
term_evals = 10)
# best performing hyperparameter configuration
instance$result
#> cp minbucket minsplit learner_param_vals x_domain classif.ce
#> <num> <num> <num> <list> <list> <num>
#> 1: -9.21034 1 44.08528 <list[4]> <list[3]> 0.04347826
# all evaluated hyperparameter configuration
as.data.table(instance$archive)
#> cp minbucket minsplit classif.ce runtime_learners
#> <num> <num> <num> <num> <num>
#> 1: -8.093058 1.000000 2.00000 0.05217391 0.006
#> 2: -9.210340 10.648145 46.54107 0.06086957 0.006
#> 3: -2.302585 1.000000 128.00000 0.06086957 0.006
#> 4: -8.146263 28.083484 90.18488 0.06086957 0.006
#> 5: -5.501698 11.868902 13.80173 0.06086957 0.006
#> 6: -7.999490 6.263489 122.64745 0.06086957 0.006
#> 7: -9.210340 64.000000 92.97889 0.11304348 0.006
#> 8: -9.210340 1.000000 44.08528 0.04347826 0.006
#> 9: -4.730547 1.000000 86.76723 0.04347826 0.006
#> 10: -9.210340 1.000000 2.00000 0.05217391 0.006
#> timestamp warnings errors x_domain batch_nr resample_result
#> <POSc> <int> <int> <list> <int> <list>
#> 1: 2025-07-10 06:37:31 0 0 <list[3]> 1 <ResampleResult>
#> 2: 2025-07-10 06:37:31 0 0 <list[3]> 2 <ResampleResult>
#> 3: 2025-07-10 06:37:31 0 0 <list[3]> 3 <ResampleResult>
#> 4: 2025-07-10 06:37:31 0 0 <list[3]> 4 <ResampleResult>
#> 5: 2025-07-10 06:37:31 0 0 <list[3]> 5 <ResampleResult>
#> 6: 2025-07-10 06:37:32 0 0 <list[3]> 6 <ResampleResult>
#> 7: 2025-07-10 06:37:32 0 0 <list[3]> 7 <ResampleResult>
#> 8: 2025-07-10 06:37:32 0 0 <list[3]> 8 <ResampleResult>
#> 9: 2025-07-10 06:37:32 0 0 <list[3]> 9 <ResampleResult>
#> 10: 2025-07-10 06:37:32 0 0 <list[3]> 10 <ResampleResult>
# fit final model on complete data set
learner$param_set$values = instance$result_learner_param_vals
learner$train(tsk("penguins"))